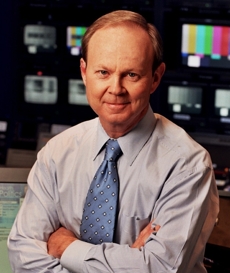
By MIKE MAGEE
In his book, “The Age of Diminished Expectations” (MIT Press/1994), Nobel Prize winner, Paul Krugman, famously wrote, “Productivity isn’t everything, but in the long run it is almost everything.”
A year earlier, psychologist Karl E. Weich from the University of Michigan penned the term “sensemaking” based on his belief that the human mind was in fact the engine of productivity, and functioned like a biological computer which “receives input, processes the information, and delivers an output.”
But comparing the human brain to a computer was not exactly a complement back then. For example, 1n 1994, Krugman’s MIT colleague, economist Erik Brynjolfsson coined the term “Productivity Paradox” stating “An important question that has been debated for almost a decade is whether computers contribute to productivity growth.”
Now three decades later, both Krugman (via MIT to Princeton to CCNY) and Brynjolfsson (via Harvard to MIT to Stanford Institute for Human-Centered AI) remain in the center of the generative AI debate, as they serve together as research associates at the National Bureau of Economic Research (NBER) and attempt to “make sense” of our most recent scientific and technologic breakthroughs.
Not surprisingly, Medical AI (mAI), has been front and center. In November, 2023, Brynjolfsson teamed up with fellow West Coaster, Robert M. Wachter, on a JAMA Opinion piece titled “Will Generative Artificial Intelligence Deliver on Its Promise in Health Care?”
Dr. Wachter, the Chair of Medicine at UC San Francisco, coined his own ground-breaking term in 1996 – “hospitalist.” Considered the father of the field, he has long had an interest in the interface between computers and institutions of health care.
In his 2014 New York Times bestseller, “The Digital Doctor: Hope, Hype, and Harm at the Dawn of Medicine’s Computer Age” he wrote, “We need to recognize that computers in healthcare don’t simply replace my doctor’s scrawl with Helvetica 12. Instead, they transform the work, the people who do it, and their relationships with each other and with patients.”
What Brynjolfsson and Wachter share in common is a sense of humility and realism when it comes to the history of systemic underperformance at the intersection of technology and health care.
They begin their 2023 JAMA commentary this way, “History has shown that general purpose technologies often fail to deliver their promised benefits for many years (‘the productivity paradox of information technology’). Health care has several attributes that make the successful deployment of new technologies even more difficult than in other industries; these have challenged prior efforts to implement AI and electronic health records.”
And yet, they are optimistic this time around.
Why? Primarily because of the speed and self-corrective capabilities of generative AI. As they conclude, “genAI is capable of delivering meaningful improvements in health care more rapidly than was the case with previous technologies.”
Still the “productivity paradox” is a steep hill to climb. Historically it is a byproduct of flaws in early version new technology, and status quo resistance embedded in “processes, structure, and culture” of corporate hierarchy. When it comes to preserving both power and profit, change is a threat.
As Brynjolfsson and Wachter put it diplomatically, “Humans, unfortunately, are generally unable to appreciate or implement the profound changes in organizational structure, leadership, workforce, and workflow needed to take full advantage of new technologies…overcoming the productivity paradox requires complementary innovations in the way work is performed, sometimes referred to as ‘reimagining the work.’”
How far and how fast could mAI push health care transformation in America? Three factors that favor rapid transformation this time around are improved readiness, ease of use, and opportunity for out-performance.
Readiness comes in the form of knowledge gained from the mistakes and corrective steps associated with EHR over the past two decades. A scaffolding infrastructure already exists, along with a level of adoption by physicians and nurses and patients, and the institutions where they congregate.
Ease of use is primarily a function of mAI being localized to software rather than requiring expensive, regulatory laden hardware devices. The new tools are “remarkably easy to use,” “require relatively little expertise,” and are “dispassionate and self-correcting” in near real-time when they err.
Opportunity to out-perform in a system that is remarkably inefficient, inequitable, often inaccessible and ineffective, has been obvious for some time. Minorities, women, infants, rural populations, the uninsured and under-insured, and the poor and disabled are all glaringly under-served.
Unlike the power elite of America’s Medical Industrial Complex, mAI is open-minded and not inherently resistant to change.
Multimodal, large language, self learning mAI is limited by only one thing – data. And we are literally the source of that data. Access to us – each of us and all of us – is what is missing.
What would you, as one of the 333 million U.S. citizens in the U.S., expect to offer in return for universal health insurance and reliable access to high quality basic health care services?
Would you be willing to provide full and complete de-identified access to all of your vital signs, lab results, diagnoses, external and internal images, treatment schedules, follow-up exams, clinical notes, and genomics?
Here’s what mAI might conclude in response to our collective data:
- It is far less expensive to pay for universal coverage than pay for the emergent care of the uninsured.
- Prior algorithms have been riddled with bias and inequity.
- Unacceptable variance in outcomes, especially for women and infants, plague some geographic regions of the nation.
- The manning table for non-clinical healthcare workers is unnecessarily large, and could easily be cut in half by simplifying and automating customer service interfaces and billing standards.
- Direct to Consumer marketing of pharmaceuticals and medical devices is wasteful, confusing, and no longer necessary or beneficial.
- Most health prevention and maintenance may now be personalized, community-based, and home-centered.
- Abundant new discoveries, and their value to society, will largely be able to be validated as worthy of investment (or not) in real time.
- Fraudulent and ineffective practices and therapies, and opaque profit sharing and kickbacks, are now able to be exposed and addressed.
- Medical education will now be continuous and require increasingly curious and nimble leaders comfortable with machine learning techniques.
- U.S. performance by multiple measures, against other developed nations, will be visible in real time to all.
The collective impact on the nation’s economy will be positive and measurable. As Paul Krugman wrote thirty years ago, “A country’s ability to improve its standard of living over time depends almost entirely on its ability to raise its output per worker.”
As it turns out, health data for health coverage makes “good sense” and would be a pretty good bargain for all Americans.
Mike Magee MD is a Medical Historian and regular contributor to THCB. He is the author of CODE BLUE: Inside America’s Medical Industrial Complex (Grove/2020).